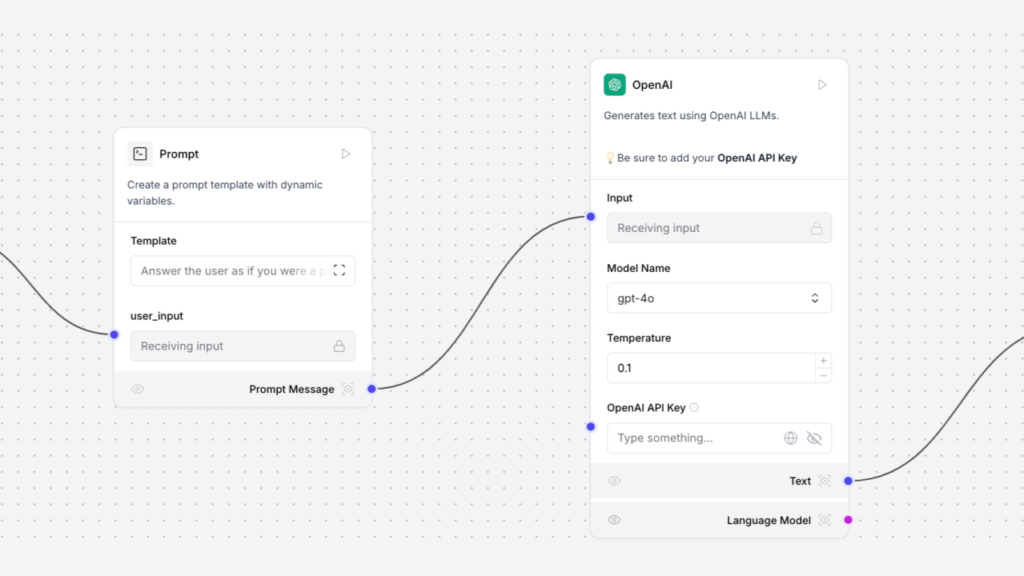
A snapshot of an AI workflow modeled in Langflow, an open tool for moderating LLM-system workflows. Image source: Langflow Docs.
As Information Architects, it has been fascinating watching our collective understanding of generative AI improve over the last few years, myself included. Openly accessible models*, frameworks and datasets, available via Ollama and Huggingface, have enabled thousands of people all over the world to get hands-on experience with these models.
The Power of Knowledge Graphs
This hands-on experience has led to broader awareness of all the variables that go into deploying a large language model to a given use case.
How does the output of an LLM vary when you use models of different token amounts and sizes?
How does the output change when you mediate your prompts with structured embeddings?
We learned about Retrieval Augmented Generation (RAG) which are software frameworks that allow LLMs to retrieve and consult structured data when answering prompts, improving accuracy and consistency. Many have learned about knowledge graphs and the Semantic Web and saw how powerful these technologies could be when applied to Retrieval Augmented Generation frameworks. Others have found that generative models have the potential to reduce the cost barriers in knowledge graph creation as well.
The Power of Linked Data
While it’s invigorating to see more people discovering the power of knowledge graphs, what I have yet to hear discussed is the state of Linked Data, which is the use of semantic web technologies to interconnect knowledge graphs. I have attended many talks about LLMs and RAG frameworks. And while I hear a lot about the power of knowledge graphs and linked data, the only examples I see used in these presentations are DBPedia or Wikidata.
To be honest, if pressed, I would not do better. I, personally, am only aware of the Linked Open Data Cloud. Of which, the largest, richest and most visible linked data sets are from DBPedia.
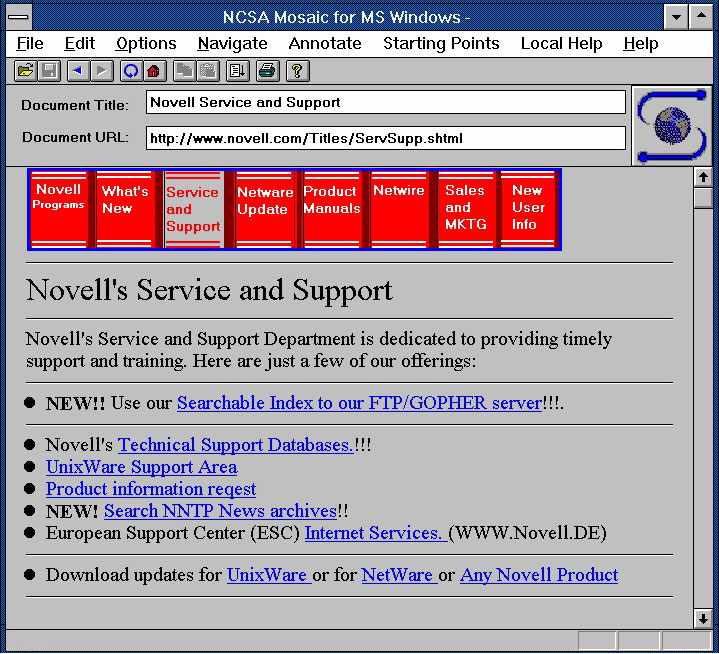
A snapshot of NSCA Mosaic, the first widely available web browser for the public, running on Windows 3.1. Source: Commonsense Design
Here Comes Everybody
The state of Linked Data reminds me a lot of the Web in 1993: an information-sharing protocol used largely by academics and corporate enterprises, initially inscrutable to the broader general public…that it is about to be subsumed by.
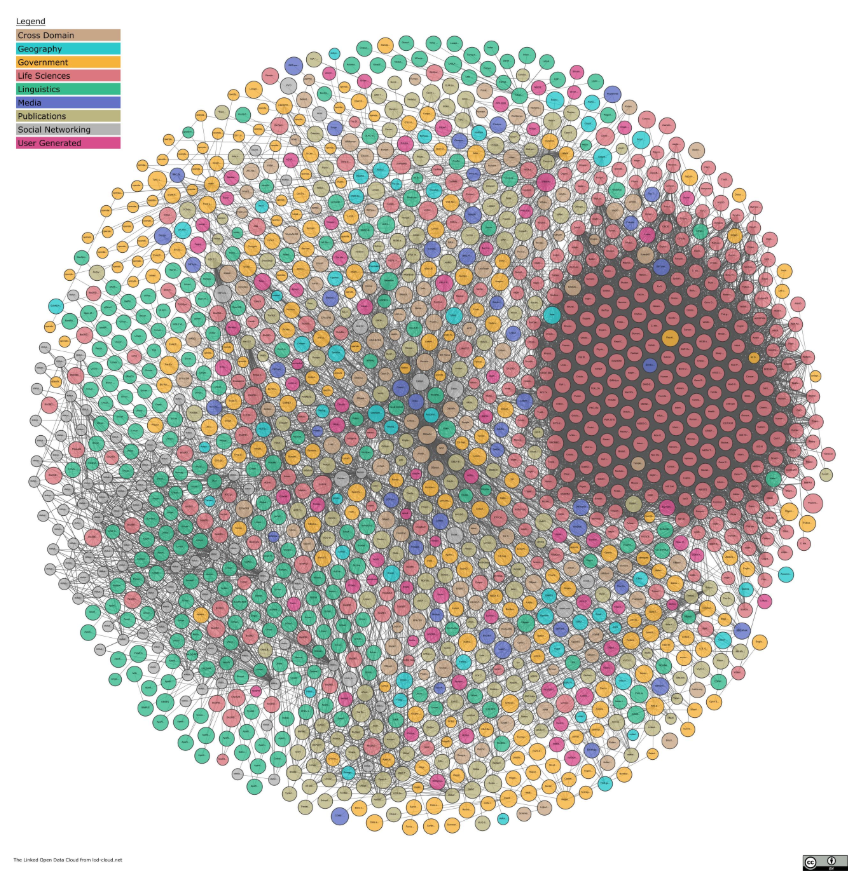
A snapshot of the Linked Open Data Cloud
What’s Next?
I want to take this moment to check in with my colleagues and the rest of the field.
As freely accessible Generative AI models and Retrieval Augment Generation frameworks begin to disseminate, where are we in our understanding of Linked Data?
As broader awareness of knowledge graphs continues to grow, how can we, as Information Architects, take advantage of this moment?
Specifically, we are at a moment where the policies and best practices surrounding Generative AI, RAG and Agentic AI are still being written. This is a timely opportunity to codify the principles of Linked Data into the minds of Generative AI developers and users. Additionally, this model can serve as the reference model for legal criteria in Trustworthy Generative AI policy.
With Linked Data as a shared mental model, people can universally refer to the same sources when discussing the design of a RAG system. As Transparent AI systems are developed, logical chains can be ascribed to specific conceptual URI, helping us to better understand Generative AI outputs.
Linked Data can also serve as a model for people to understand what a given Generative AI implementation has been trained on. Imagine if there were a Linked Data Facts Label for every generative AI implementation, that outlined what models and linked data sources are considered. While I am being a bit facetious about the format, a summary of data sources and models could go a long way to improving generative AI accuracy, consistency, transparency and explainability.
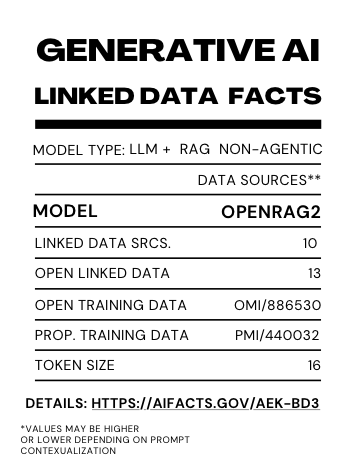
A hastily mocked-up example of information that can be provided to users of generative AI models. Expanding this information gives you specific URI information for linked data sources, along with training information (if available)
Beyond the Linked Open Data Cloud, are there gaps in my understanding of the current state of the Linked Open Data ecosystem that I am missing?
It seems that the potential for Linked Data is largely going undiscussed in Generative AI and Retrieval Augmented Generation communities. At the risk of drawing attention to my own ignorance, I wanted to put out a call for more visibility on this topic, specifically with an eye toward the Information Architecture profession. Given the number of times I have seen DBPedia and Wikidata used as placeholders for Linked Data, I don’t think I am alone.
* I say “Openly accessible models” to state that these models are openly accessible to the public. They are not open in the same sense as open-source software. The definition of open-source models is in flux, but I would refer you to the Open Source Initiative’s Open Source AI Definition 1.0. As of the time of this writing, none of the “open” models meet this definition.
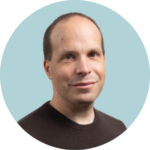